728x90
import tensorflow as tf
import pandas as pd
import numpy as np
from sklearn.model_selection import train_test_split
from sklearn.impute import SimpleImputer
from tensorflow.keras.layers.experimental.preprocessing import TextVectorization
import tensorflow_hub as hub
import matplotlib.pyplot as plt
import random
# Load and preprocess data
df = pd.read_csv(
"/kaggle/input/ml-olympiad-can-you-guess-beer-style/train.csv"
)
# Select columns
df = df[
[
"Description",
"ABV",
"Min IBU",
"Max IBU",
"Astringency",
"Body",
"Alcohol",
"Bitter",
"Sweet",
"Sour",
"Salty",
"Fruits",
"Hoppy",
"Spices",
"Malty",
"review_aroma",
"review_appearance",
"review_palate",
"review_taste",
"review_overall",
"number_of_reviews",
"Style",
]
]
# Select numeric columns only
numeric_cols = df.select_dtypes(include=np.number).columns.tolist()
# Fill NaN for numeric columns
imp_mean = SimpleImputer(missing_values=np.nan, strategy="median")
df[numeric_cols] = imp_mean.fit_transform(df[numeric_cols])
# For non-numeric columns (in this case "Description"), you could fill with an empty string or some other strategy
df["Description"].fillna("", inplace=True)
# Split dataset
train_set, val_set, test_set = np.split(
df.sample(frac=1, random_state=42),
[int(len(df) * 0.8), int(len(df) * 0.9)],
)
# Process the description
vectorizer = TextVectorization(max_tokens=5000, output_sequence_length=200)
train_text = train_set['Description']
vectorizer.adapt(train_text.values)
# Create datasets
def df_to_dataset(dataframe, shuffle=True, batch_size=32):
dataframe = dataframe.copy()
labels = dataframe.pop('Style')
ds = tf.data.Dataset.from_tensor_slices((dict(dataframe), labels))
if shuffle:
ds = ds.shuffle(buffer_size=len(dataframe), seed=42)
ds = ds.batch(batch_size)
ds = ds.prefetch(batch_size)
return ds
train_ds = df_to_dataset(train_set)
val_ds = df_to_dataset(val_set)
test_ds = df_to_dataset(test_set)
# Define model
def create_model():
# text input branch
text_input = tf.keras.Input(shape=(), dtype=tf.string, name='Description')
x = vectorizer(text_input)
x = tf.keras.layers.Embedding(
input_dim=5000, output_dim=64, mask_zero=True)(x)
x = tf.keras.layers.Bidirectional(tf.keras.layers.LSTM(64))(x)
x = tf.keras.layers.Dropout(0.2)(x)
x = tf.keras.layers.Dense(64, activation='relu')(x)
text_output = tf.keras.layers.Dense(64, activation='relu')(x)
# numeric input branch
numeric_inputs = [tf.keras.Input(shape=(1,), name=c) for c in train_set.drop(columns=['Description', 'Style']).columns]
x = tf.keras.layers.concatenate(numeric_inputs)
x = tf.keras.layers.Dense(64, activation='relu')(x)
x = tf.keras.layers.Dropout(0.2)(x)
numeric_output = tf.keras.layers.Dense(64, activation='relu')(x)
# concatenate text and numeric branches
concatenated = tf.keras.layers.concatenate([text_output, numeric_output])
output = tf.keras.layers.Dense(len(df['Style'].unique()), activation='softmax')(concatenated)
model = tf.keras.models.Model([text_input] + numeric_inputs, output)
model.compile(optimizer='adam',
loss='sparse_categorical_crossentropy',
metrics=['accuracy'])
return model
# Train the model
model = create_model()
history = model.fit(train_ds, validation_data=val_ds, epochs=10, callbacks=[
tf.keras.callbacks.EarlyStopping(patience=3, restore_best_weights=True)])
# Evaluate the model
model.evaluate(test_ds)
# Visualize training history
plt.plot(history.history['loss'])
plt.plot(history.history['val_loss'])
plt.title('Model Loss')
plt.ylabel('Loss')
plt.xlabel('Epoch')
plt.legend(['Train', 'Val'], loc='upper right')
plt.show()
plt.plot(history.history['accuracy'])
plt.plot(history.history['val_accuracy'])
plt.title('Model Accuracy')
plt.ylabel('Accuracy')
plt.xlabel('Epoch')
plt.legend(['Train', 'Val'], loc='upper left')
plt.show()
Epoch 1/10
13/13 [==============================] - 21s 432ms/step - loss: 257.0126 - accuracy: 0.0507 - val_loss: 151.4959 - val_accuracy: 0.0000e+00
Epoch 2/10
13/13 [==============================] - 3s 216ms/step - loss: 156.8023 - accuracy: 0.0628 - val_loss: 121.1608 - val_accuracy: 0.0192
Epoch 3/10
13/13 [==============================] - 3s 207ms/step - loss: 119.4563 - accuracy: 0.1039 - val_loss: 103.4732 - val_accuracy: 0.0385
Epoch 4/10
13/13 [==============================] - 2s 183ms/step - loss: 101.0891 - accuracy: 0.0870 - val_loss: 95.3936 - val_accuracy: 0.0769
Epoch 5/10
13/13 [==============================] - 2s 178ms/step - loss: 87.5844 - accuracy: 0.1014 - val_loss: 98.3966 - val_accuracy: 0.0962
Epoch 6/10
13/13 [==============================] - 2s 146ms/step - loss: 80.8935 - accuracy: 0.1014 - val_loss: 96.4117 - val_accuracy: 0.0577
Epoch 7/10
13/13 [==============================] - 3s 200ms/step - loss: 64.1204 - accuracy: 0.1111 - val_loss: 92.1796 - val_accuracy: 0.1346
Epoch 8/10
13/13 [==============================] - 2s 121ms/step - loss: 64.1571 - accuracy: 0.1473 - val_loss: 87.7057 - val_accuracy: 0.1154
Epoch 9/10
13/13 [==============================] - 2s 149ms/step - loss: 60.5621 - accuracy: 0.1184 - val_loss: 92.3790 - val_accuracy: 0.0769
Epoch 10/10
13/13 [==============================] - 2s 130ms/step - loss: 49.4580 - accuracy: 0.1449 - val_loss: 98.1194 - val_accuracy: 0.0962
2/2 [==============================] - 0s 16ms/step - loss: 52.7078 - accuracy: 0.1538
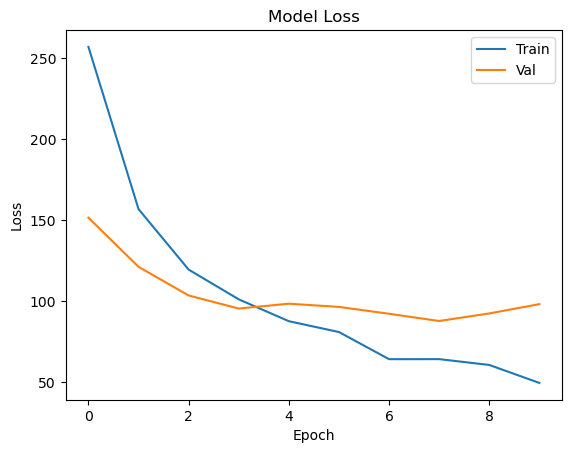
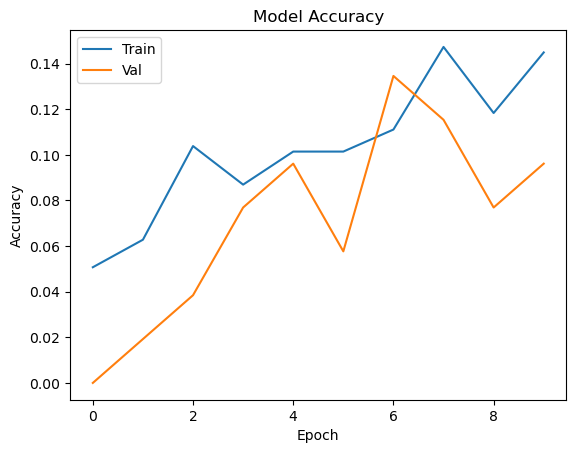
In [2]:
# Assuming `test_set` is your test dataset
preds = model.predict(df_to_dataset(test_set, shuffle=False, batch_size=1))
52/52 [==============================] - 3s 8ms/step
In [3]:
# Create DataFrame for submission
submission_df = pd.DataFrame()
submission_df['ID'] = test_set.index # Use index as 'ID'
submission_df['Style'] = tf.argmax(preds, axis=-1).numpy() # Assign predictions to 'Style' column
# Save DataFrame to csv file
submission_df.to_csv('submission.csv', index=False)
728x90
'강얼쥐와 함께 즐겁게 읽는 AI' 카테고리의 다른 글
사람들은 복잡한 기계를 다룰 때에는 설명서가 필요하다고 생각하면서 이상하게도 우주에서 가장 복잡한 기계 중 하나인 본인의 뇌를 사용할 때는 어떠한 설명서도 필요 없다고 착각한다 (0) | 2023.12.04 |
---|---|
Attention Is All You Need (2) | 2023.06.23 |
구글은 멍청했습니다. (0) | 2023.06.02 |
가끔 chat gpt가 뭐가 그리 대단한지 잘 모르겠다는 사람들을 소수 만난다 (0) | 2023.05.27 |
미쳤따리 (0) | 2023.05.14 |